Yu Chen
PhD candidate and Early-Stage-Researcher
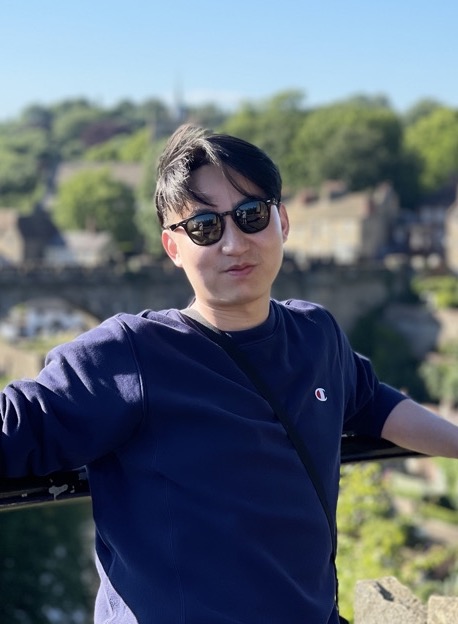
Univ of Liverpool
Isterre, Université Grenoble Alpes
I’m currently a postdoctoral research associate at Institute for Risk and Unceratinty, University of Liverpool, working at an intersection of uncertainty quantification and deep learning with a focus on probability bounds analysis.
I’ve got my PhD on the subject of Modeling and dealing with vague and sparse information using Machine Learning, **funded by the EU-Horizon 2020 & Marie Skłodowska-Curie Actions project URBASIS as an ESR.
During my PhD, I am dedicated to developing robust Deep Learning-based computational frameworks against data problems (imprecise, limited, scarce, imbalanced or OOD data), and propagating associated uncertainty through computational probabilistic models in an efficient way. My contribution revolves around two aspects: (I)
equiping DL models with uncertainty awareness, allowing for robustness; (II)
incorporating DL with prior (physical) domain knowledge, allowing for fusion of knowledge.
Research interests
1
2
3
4
5
6
- **{Bayesian, knowledge-informed, Evidential, generative}** Deep Learning
- Stochastic modeling of uncertainties in engineering
- robustness of ML against data problems
- Imprecise probability
- probability bounds analysis
- Risk-based optimal decision making and cost-benefit analysis
news
2024 |
October
|
---|---|
2023 |
Nov
|
2022 |
Sep
|
2021 |
June
|